Breadcrumb navigation
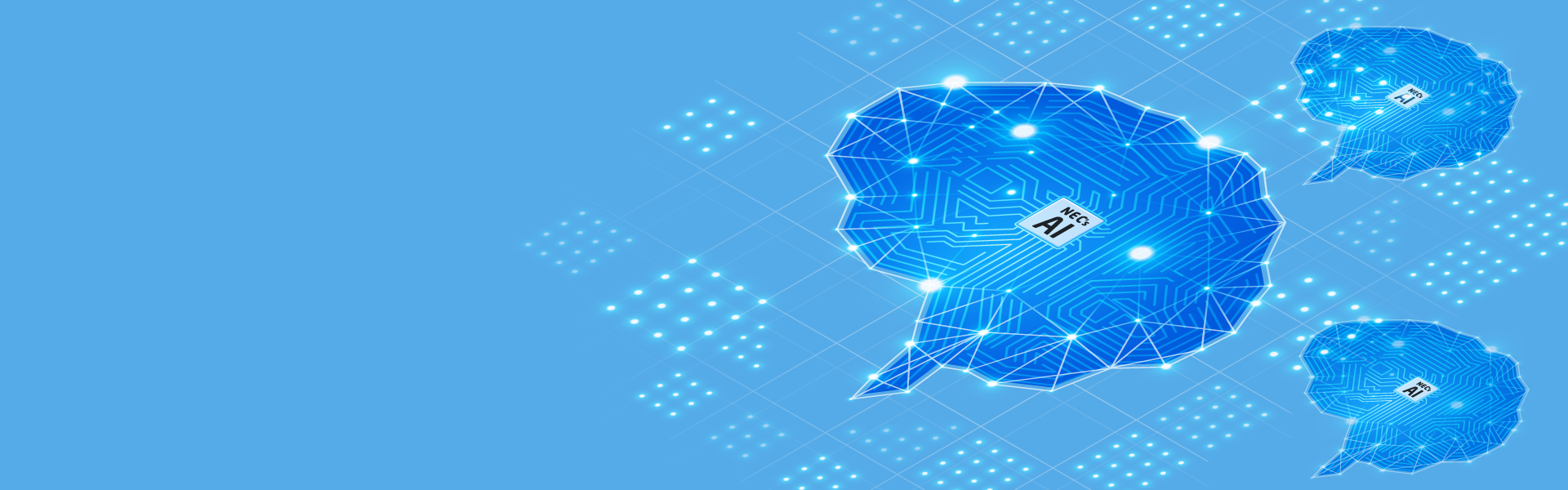
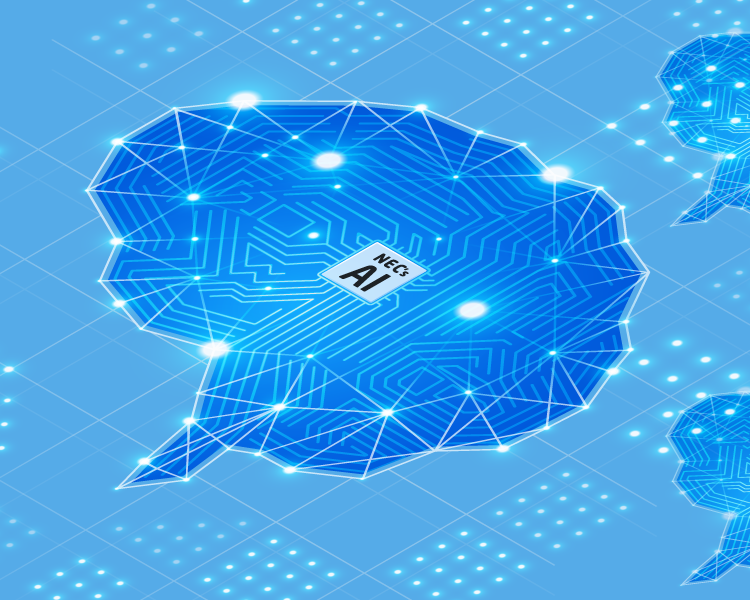
AI
Our state-of-the-art AI leverages the latest advancements in deep machine learning to deliver unparalleled performance and precision. By incorporating cutting-edge algorithms and data processing techniques and proprietary datasets, our AI systems set a new standard for innovation in the industry.
State-of-the-Art Proprietary AI
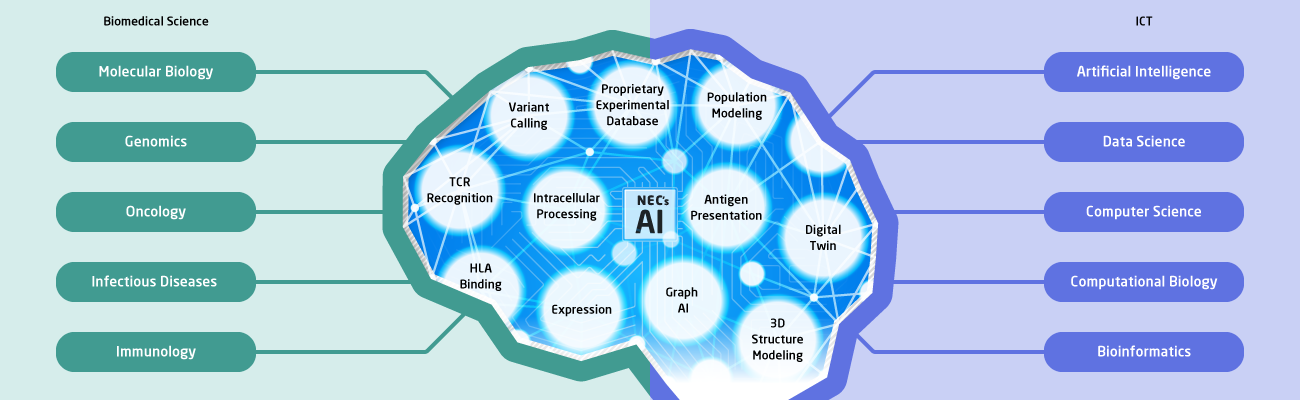
Graph AI
Graph AI is a revolutionary technology that facilitates highly accurate learning by utilizing knowledge graphs within data sets, including those containing defects—a common occurrence in biotechnology. This technology is broadly applicable across a wide range of fields.
Link:Graph-based Relational Learning:
NEC Technical Journal | NEC
BERTMHC
In designing personalized vaccines, we aim to predict the likelihood of an immune response based on an individual's MHC alleles. We focus on developing models to predict MHC class II peptide binding and presentation, using self-supervised pretraining on large protein sequence datasets. Our work improves prediction accuracy and has potential applications in T-cell-based immunotherapy, offering new directions for training models in peptide binding and presentation.
NeoMutate
The complex characteristics of cancer tissue, particularly tumor genetic intra-heterogeneity, combined with sequencing and alignment artifacts make somatic variant calling a challenging task. To address this, we developed the NeoMutate framework, which integrates seven supervised machine learning algorithms to leverage the strengths of multiple variant callers based on a unique set of biological and sequence features.
Digital Twin
We developed a digital twin simulation using a database of HLA haplotypes from approximately 22,000 individuals to model the effectiveness of different epitope hotspots in a diverse population. This method identifies the optimal set of hotspots for maximum global coverage. By integrating the NEC Immune Profiler's predictions on antigen presentation, cell surface binding, and immunogenicity with a robust Monte Carlo and digital twin simulation, we pinpoint a subset of epitope hotspots for potential use in broadly protective vaccine formulations.
Why can we develop cutting-edge AI?
NEC Bio benefits from the extensive expertise of the larger NEC Group, a renowned leader in ICT and AI technologies. Our cutting-edge AI is developed by leading experts and pioneers in machine learning. Learn more about our leaders by exploring our AI expert panel.
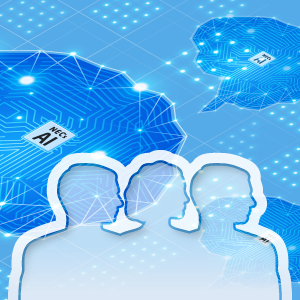